31 - Sinogram-based data augmentation to automate the non-destructive control of mechanical parts
Abstract
Cetim Sud-Ouest (CSO), a subsidiary of the CETIM, the French technical center for mechanical industries is a specialist in X-ray computed tomography (XCT) and metrology. CETIM is a leader in major industrial challenges and a pillar for innovation. Additive manufacturing (AM) using metallic powders has become suitable not only for prototyping but also for producing complex shapes. CSO is willing to characterize porosities which are typical defects regardless of the processes of this technology. Non-destructive techniques (NDT) via XCT is a key point to provide three-dimensional internal information on AM samples of various alloys. XCT produces X-ray profiles, called sinograms, used to reconstruct the volume. Our dataset is composed of these raw data. First, a neural network (NN) classification is performed. To collect dimensional information, segmentation based on NN is forecast. Deep learning (DL) requires thousands of data. It is not feasible as XCT scans are time-consuming and costly. There is a need to augment our initial dataset composed of sinograms with artificial realistic ones. DL will estimate dimension parameters of defects using this representative dataset. To rule out discretisation artifacts, ill-posed problems and approximate reconstruction of voxel, sinograms are favoured. They can be interpreted in different ways from an image through contours and edges of meaningful markers to the mathematical approach as it is a sum of sinuses. A methodology has been set up to extract and/or add defect signatures into them to enrich the dataset in addition to the simulations generated. Rotations and translations can be operated in the Radon space by applying a sinus function to the image to modify amplitude and phase. Feature detection using 2D filters in the image emphasises the main sinuses that are related to edges in the reconstructed image. These sinusoid-like curve decomposition provide the spatial representation of key objects. Using the additivity of the Radon transform, some sinuses can be added or removed leading to an augmentation of the representation. New sinograms are created from the ones with significant real defects. By combining a spatial representation and an intensity map inferred by a generative adversarial network (GAN), we could obtain a realistic sinogram bypassing the inverse problem solving. The network can learn on a substantial dataset with a good bias-variance tradeoff. Our study gives promising outcomes by giving a new methodology to create realistic sinograms. This data augmentation could lead to NN for more accurate training of AM part defects.
Speaker
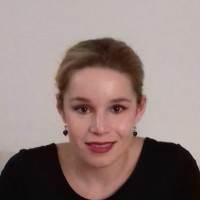
Nina Lassalle-Astis
Discover speaker profileCETIM Sud-Ouest, Pau, France / LaBRI, Talence, France
See LinkedIn profileConference
31 - Sinogram-based data augmentation to automate the non-destructive control of mechanical parts
Date/Time
20/03/2024
11:55 am -12:15 pm
Location
Room 6